We are thrilled to announce that a paper led by our company has been accepted for the second consecutive year at NeurIPS, the world's top machine learning (ML) conference. At NeurIPS 2023, over 3,000 submissions were made from Japan alone, but only about 100 passed the rigorous review process. It's extremely rare for a bio-tech startup like ours to be selected. We are deeply grateful to our business partners, grant providers, investors, collaborative research partners such as Sakura Internet, and all our supporters for their continued encouragement and backing.
This achievement demonstrates the potential of our proprietary "Antigen-Labeled Antibody Big Data" and the possibilities of applying language models. Special thanks go to our ML and tech lead, Mr. Tsuruta, our MLOps lead, Mr. Tamura, and all the researchers of our small startup who crossed the boundary between biology and IT to work together.
There are three key themes that have become possible for the first time through the synergy of our bio dataset and computational science:
- Cellular Level: Distinguishing between "normal and abnormal cells."
- Molecular Level: Predicting antibody-antigen pairings by matching amino acid sequences.
- Atomic Level: Selecting antibodies that bind to specific 3D structures.
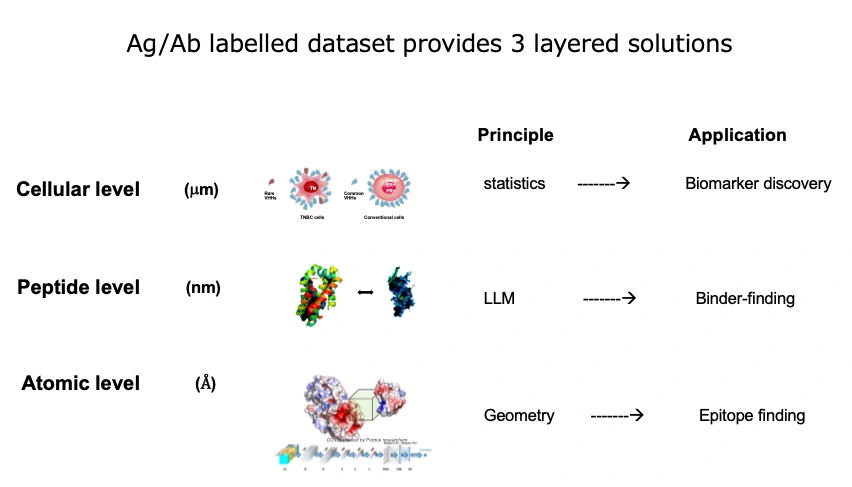
Using antibody data requires advanced mathematical techniques and vast computational power. Until now, large datasets pairing antigens and antibodies were rare, and there was little precedent for the necessary data processing. COGNANO is the first in the world to internally produce such big data, package it by MLOps, and store it in the cloud. With this setup, we are tackling these three challenges. The paper accepted at NeurIPS addresses theme 2. Incidentally, theme 1 has already produced results, such as identifying new target molecules for difficult-to-treat cancers. We plan to collaborate with IT tech to develop theme 3 in the future. Since theme 3 begins with understanding 3D structures, collaboration with algorithms like AlphaFold will be key. The IT industry often envisions theme 3 when thinking about antigen-antibody datasets.
Here are a few examples of practical applications:
- Discovering unknown biomarkers, enabling early diagnosis and drug development for detecting and treating cancer.
- Rapidly responding to mutating viruses and discovering preventive drugs from a library of solutions.
- Using machines to design drugs that are difficult to create with traditional methods by targeting unique structural motifs.
We believe that within 10 years, new drugs will be partially predicted by AI. For details on the paper that was accepted this time, please refer to Tsuruta’s blog post. From a bio-researcher's perspective, I’d like to offer some thoughts on how AI-driven drug discovery will progress.
A good example to refer to here is ChatGPT. Regardless of what model you build, machine learning (ML) requires a vast amount of data to succeed. The brilliance of Altman’s team at OpenAI, which brought ChatGPT to success, lies in breaking through the uncertainty of how much data needs to be fed before the machine starts giving coherent answers. OpenAI must have needed enormous computational resources, and the competition for funding must have been fierce.
A key challenge for the bio industry is whether there is a comparable amount of data as was fed into ChatGPT. The answer is no. AlphaFold is a well-known success story of bio-related ML, but it was built by training an algorithm with hundreds of thousands of protein structure datasets, which are highly regular and contextually clear. However, in drug discovery, we don’t just want to know protein structures. Drugs work by binding to target molecules, so understanding molecular interactions is crucial—but there is a significant lack of data in this field.
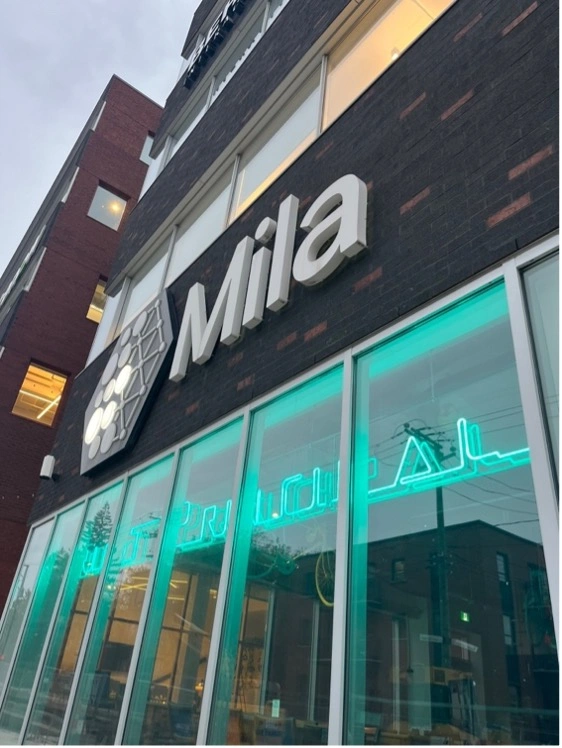
Antibody-antigen binding, as demonstrated through bio-experiments, provides clear labeled data with binary results (binding or not). Our paper has successfully demonstrated COGNANO’s concept for two consecutive years. In theme 3 of AI-driven drug discovery, we also believe that high-quality data will be crucial. The impact of dataset quality on ML training efficiency has been demonstrated in many studies. For more information on these studies, please see Tsuruta’s essay.
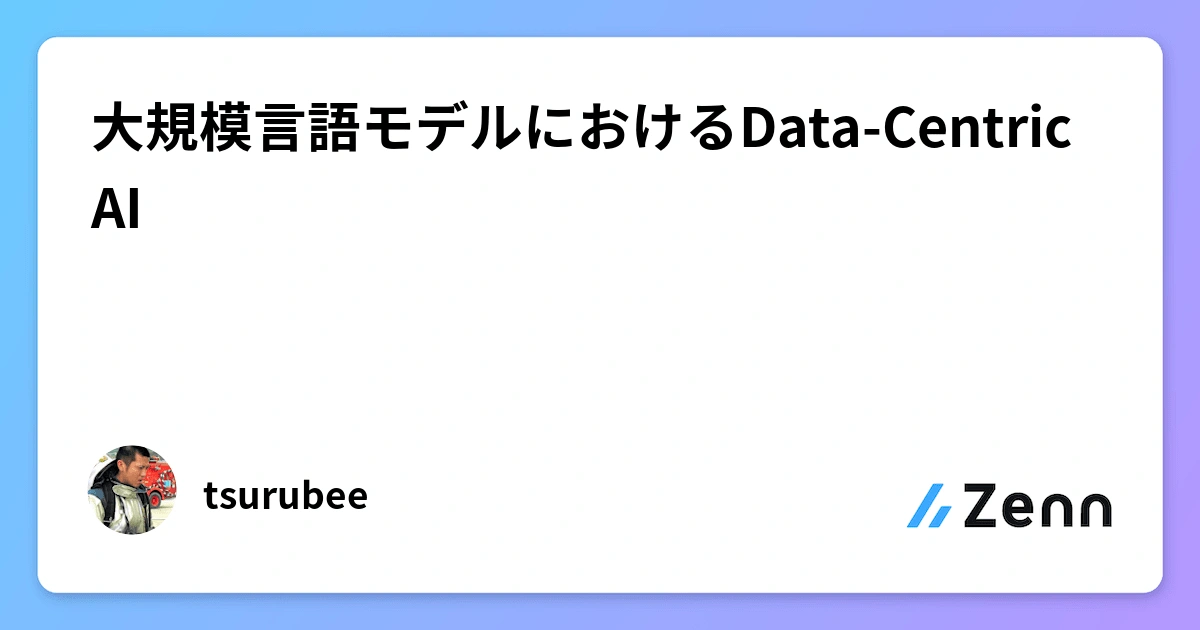
The high-quality labeled data that COGNANO—powered by alpacas—produces could be the key to winning the AI drug discovery race. In fact, more teams from the IT industry are showing interest in COGNANO’s data, hoping for collaborative research. After pitching at London Tech Week (LTW) this past June, we received an enthusiastic response, with several teams from the UK visiting us afterward. For ML engineers, the direction COGNANO is taking in AI-driven drug discovery is easy to grasp. On the other hand, we’ve seen growing interest from the bio industry, with many noting that we are doing something exciting. We will continue to share COGNANO’s achievements through future paper publications and patent filings.
Personally, I’m excited about how new data generated from cutting-edge technologies can accelerate ML. In traditional bio-research, only "the very best data" was published, while 99.99% of the data remained unused. Now, thanks to ML, we can use all of it—nothing needs to be wasted. I’m grateful for this shift, which suggests a major industrial change. The inefficiency of bio-research was a primary reason it could only thrive in academia. From an industry perspective, inefficient basic research was left to universities, while the profit-driven aspects were handled by businesses. However, when bio foundational research transforms into data science, there is potential for it to become a viable business. This also means that data, which previously held little value, can now determine success. Just as crude oil, once seen as a troublesome liquid, suddenly became valuable with the invention of the gas-engine, we can understand why AlphaFold has garnered so much attention. The question now is: which bio-data should be produced, and which teams should join to win the first AI-driven drug discovery race in human history? Stay tuned for more updates from COGNANO!