It's already the end of the year. COGNANO achieved a steady growth this year, too. Thank you everyone!
Most of the topics were Large Language Models (LLM) such as ChatGPT, and while sessions such as self-driving car algorithms and global environmental energy were also the mainstream, about 10% of the participants were related to biology.
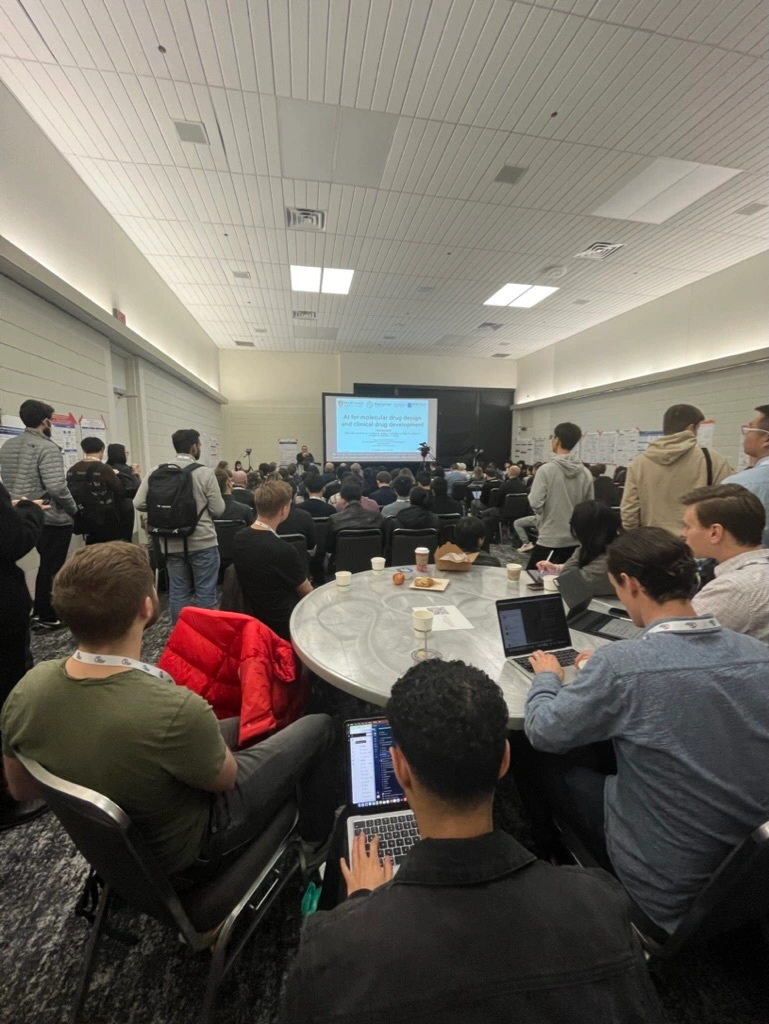
The 15,000 participants, in addition to Big Tech programmers and researchers such as Amazon, Microsoft and and Google, there were many participants from famous universities (MIT, Stanford, Harvard, UC Berkeley, UCLA, Montreal MILA). There were hardly any Japanese people. While most of the language model announcements are influenced by the success of ChatGPT, Big Tech is also expanding into bio fields and is very motivated. It seems like they are making a huge investment in the promising bio-business and actively hiring human resources. IT techs cannot create real biodata in-house, so they have no choice but to start from open databases. There is an increasing number of drug finding trials such as AlphaFold and Rosetta with a tendency to research focused on chemical fitting to 3D proteins. This approach is theoretically feasible, but being an expert in biology, I anticipate how difficult it is to predict a new drug by this method because chemicals are not immune from unexpected off-target effects, or potential side effects.
Chemicals have a background in which side effects are difficult to predict, and No wonder the odds of a given compound being approved as a new drug is as low as 0.04%, no better than those of hitting the jackpot in a lottery. Therefore, it is understandable that 3D-prediction AI would save time and money compared to screening large amounts of real substances in the laboratory. Meanwhile, a limited number of global biotech companies were participating, among them was Astra Zeneca who set up a booth.
There were no large-scale datasets on antigen-antibody labels similar to COGNANO in the whole conference. Presenters Tsuruta and Yamazaki reported on the reactions to the world's first large-scale antibody data set.
The excitement of the venue reminded me of the time when COGNANO was founded. Bioscience cannot obtain "fair" data. Life is a dynamic existence made up of multilayered phenomena. Since life is made up of countless parameters, researchers have no choice but to focus on a certain parameter and repeat the process of making a hypothesis and verifying it.
For example, suppose we have the following statistics “People who drink two or more cups of coffee a day live longer than people who drink one cup or less.”
This statistical result makes it seem like coffee is good for humans. However, it may be that “people who cannot afford to drink coffee multiple times a day have relatively short lifespans,” and the group “so weak that they cannot accept the strong aroma of coffee” may have a relatively short lifespan. It may be lowering the statistical value of longevity. Unfortunately, all too often we end up with such a simplistic announcement as “coffee contributes to longevity.” And we know well that such a catchy phrase is far more appealing to the media people.
In order to verify the hypothesis, further data analyses and intervention experiments are required. In order to avoid “phenomenology,” genetic engineering and biotechnology have been the main focus in the last 70 years. Since molecules are studied based on certain substances, it was expected that contextual errors would be reduced (making biology closer to ‘genuine’ science). Gene modification technologies such as CRISPR can uniquely estimate gene function by deleting or adding genes from living organisms.
Nowadays the current trend creates another problem. The recent advance in molecular biology has broken down the biotechnology into narrow-ranged, segmented research sections. Such trend toward more narrow-ranged research makes it harder for us to understand life as a whole. I believe researchers should be warned against the danger of the trend , but I myself have no idea what I should do about it.
A line of the lyric in a popular anime song goes off in my mind from time to time “I don't want it to end without understanding it (lyrics by Takashi Yanase).'' How do you understand? Who knows? When I was struggling for answers to these questions, I got to know IT engineers and shared data with them (perhaps won their empathy with me as well) at the AI conference. I’d say these were all timely god-sends to me. For me, participating in NeurIPS was the the moment I realized that I had become a beacon for researchers who are feeling their way through the maze of biology.
How did Alpha GO defeat human champions? I hear that a computer learned how the next move contributes to victory based on 3,000 years of game records stored at the Nihon Ki-in, and succeeded in selecting an effective "hands" that contributes to the winning rate. Since it is a board game with a clear definition of winning and losing, it is possible to train by labeling wins as 1 and losses as 0. After Dr. David Silver of DeepMind transcended the human win/loss level, he took an even more ambitious approach. He conducted an experiment to see what would happen if multiple computers played Go against each other automatically, created a new "Go record" profile, and gave new training data that was not limited to humans (as bias) to predict effective "hands". This algorithm is called Alpha GO-ZERO. Zero means no human match data is included. If the "hands" devised by masters over 3,000 years of human history are exhaustive, then Alpha GO might be competitive to Alpha GO-ZERO. On the other hand, if the "training data without human intervention" learned by Alpha GO-ZERO exceeded the accumulation of humankind over 3,000 years, the ZERO will show much superior performance... As a result of the experiment, Alpha GO-ZERO defeated Alpha GO by a landslide. From this fact, it can be presumed that the historical Go intelligence by humans was only a part of a huge mathematical space. There are vast possibilities beyond the concepts that humans have explored. This episode is a serious problem of COGNANO. How to deal with the infinite space of the life hierarchy is a tougher challenge than Go game. I have to accept my own limitation and find companions to go on this adventure with.
For the first time in history, COGNANO is creating unlimited "molecular interaction" data using alpacas, which are living data generators. This is an initiative that can be called "Alpha GO by COGNANO.'' This goal can also be said to be the fully automated drug discovery. And next, what should be Alpha GO-ZERO by COGNANO? The world of living creatures that we are currently seeing on earth is the result of a single evolution, chosen among countless possibilities, though there must have been countless other paths. Life is an entity that maintains a normal state while undergoing transitions, and its basic principle is "molecular interactions." Genes and proteins are even materials for life. Long ago, life began the moment molecules interacted. If we can understand intermolecular interactions, we can encounter "life that has never been seen'' on a computer. For example, there must be more efficient reaction of photosynthesis in the andromeda nebula by the other biological reaction. COGNANO’s dataset is a fast track to accessing the principle in "molecular interactions".
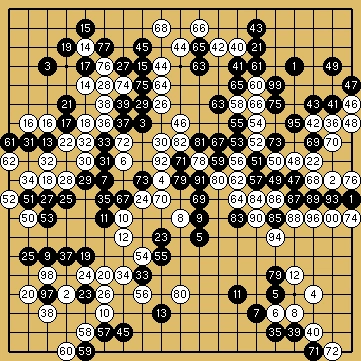
Excited over the promising prospect on our project, I lost my sense of time. The conference was coming to an end before I knew it. I left the venue vowing to return to NeurIPS 2024.
(Edited by Dr. Kimio Fujii)