Antibodies are the most important therapeutic modality in drug discovery because there is no substance that binds to target molecules (antigens) as precisely and strongly as antibodies. Living organisms can produce a huge variety of antibodies, derived from genes, in almost unlimited quantities - so much so that it is theoretically possible to search for effective antibodies based on huge collections of antibody-encoding genes in vivo. However, it is not easy to decipher them, and there are limits for data accumulation due to the complicated genes. By immunizing alpacas, which possess a simple antibody-encoding gene meaning they can produce a wide array of antibodies, COGNANO acquired a digital โlibraryโ of antibody sequences and their binding activity to different antigens. Generally, the binding between an antibody and an antigen is one-to-one correspondence, and there is only one binding site (known as the epitope). We demonstrate with this dataset that the artificial intelligence has the potential to predict the binding ability of previously unknown antibodies. We are making this dataset available for the research community as the world's largest and most precise antigen/antibody dataset, in the hope that it accelerates progress in AI enabled drug discovery. We hope that future work explores the possibility of not only predicting binding, but also identifying epitopes and the responsible amino acid sequences in both antigens and antibodies. We believe that this is an important step forward in automatic drug discovery. COGNANO will present this achievement at NeurIPS 2023 in collaboration with the Google team.
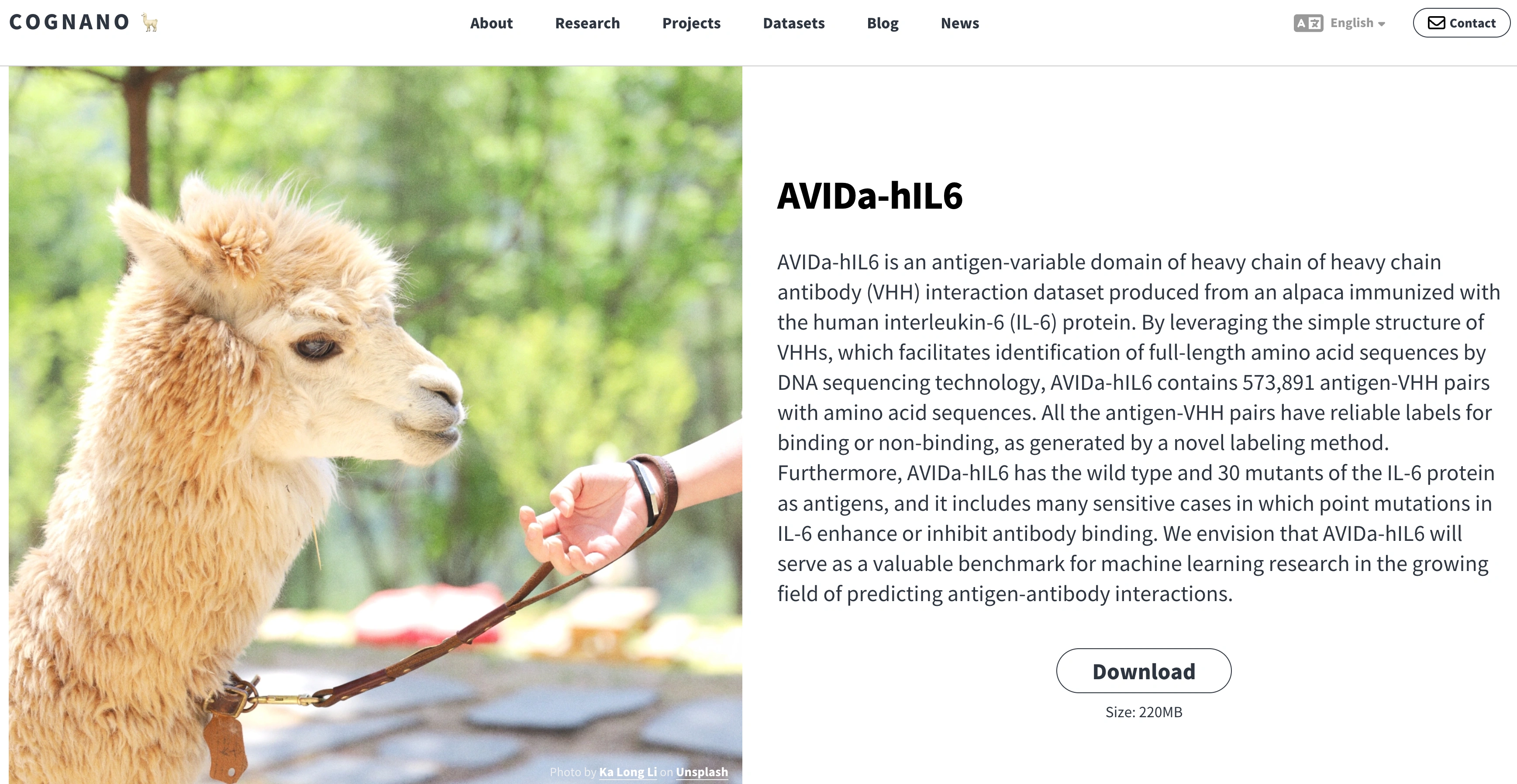
Website for downloading released dataset
Title | AVIDa-hIL6: A Large-Scale VHH Dataset Produced from an Immunized Alpaca for Predicting Antigen-Antibody Interactions |
Authors | Hirofumi Tsuruta, Hiroyuki Yamazaki, Ryota Maeda, Ryotaro Tamura, Jennifer N. Wei, Zelda Mariet, Poomarin Phloyphisut, Hidetoshi Shimokawa, Joseph R. Ledsam, Lucy Colwell, Akihiro Imura |
URL |
1. Background
Antibodies are proteins that play an essential role in the immune system. Antibodies have become an important class of therapeutic agents to treat human diseases because of their high target specificity and binding affinity. To accelerate therapeutic antibody discovery, computational methods, especially machine learning, have attracted considerable interest for predicting specific interactions between antibody candidates and target antigens such as viruses and bacteria. However, progress in therapeutic antibody discovery has lagged behind progress in other areas of drug discovery because of the lack of availability of high-quality, large-scale datasets of antigen-antibody interactions. In particular, the publicly available datasets in existing studies have notable limitations, such as small sizes and the lack of non-binding samples and exact amino acid sequences. Therefore, large-scale datasets that overcome the limitations of existing datasets are essential to further accelerate AI drug discovery.
2. Research contributions
- We release AVIDa-hIL6, which is the largest existing dataset for predicting antigen-antibody interactions (10 times larger than any other public dataset) and contains amino acid sequences of antigens and antibodies and binary labels for binding and non-binding pairs.
- We have designed a novel data generation method by using the immune system of a live alpaca. Because our data generation method is applicable to any target antigen, it can be a fundamental technology for establishing a more comprehensive database of antigen-antibody interactions. In fact, we used the same approach to generate a dataset for SARS-CoV-2 variants and successfully found effective antibodies.
- We report experimental benchmark results on AVIDa-hIL6 by using machine learning models. These results confirm that AVIDa-hIL6 provides valuable benchmarks for machine learning research in the growing field of predicting antigen-antibody interactions.
3. Released dataset (AVIDa-hIL6)
AVIDa-hIL6 is available on the website (https://avida-hil6.cognanous.com) under a CC BY-NC 4.0 license. AVIDa-hIL6 contains amino acid sequences of the human interleukin-6 (IL-6) protein used as the antigen and antibodies and binary labels for binding and non-binding pairs.
Furthermore, AVIDa-hIL6 contains information on the interaction of diverse antibodies with 30 different mutants produced by artificial point mutations, in addition to the wild-type IL-6 protein. This assumes that antigen mutants emerge one after another to evade the immune system, as in the COVID-19 pandemic. Notably, AVIDa-hIL6 contains many sensitive cases in which point mutations in the IL-6 protein enhance or inhibit antibody binding, thus providing researchers with valuable insights into the effects of antigen mutations on antibody binding.
4. Perspectives
The major limitation of AVIDa-hIL6 is the lack of antigen diversity: specifically, AVIDa-hIL6 only has the IL-6 protein as an antigen. This limitation leads to the narrow applicability of a model trained on AVIDa-hIL6. In fact, it is difficult for a machine learning model trained using only AVIDa-hIL6 to predict antibodies that are effective against antigens other than IL-6 protein. However, in drug discovery applications, there is a need to find effective antibodies against new emerging antigens.
An essential approach to overcome this limitation will be to accumulate labeled data for a wider variety of antigens and their mutants. Our data generation method has the advantage of being applicable to any target antigen. In the future, we plan to generate and release datasets for various antigens, which should be more practical for building models to predict antigen-antibody interactions.